报告题目:Machine Learning for System Operation and Application Orchestration in the IoT-Edge-Cloud Continuum
报 告 人:Raffaele Gravina副教授
报告时间:2025年4月17日 星期四下午16:00—17:00
报告地点:电子信息学科研究院会议室
腾讯会议ID:582-912-892
欢迎广大师生踊跃参加!
报告人简介
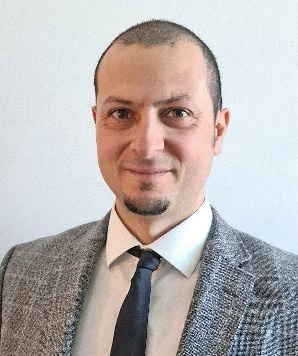
Raffaele Gravina () is Associate Professor of Computer Engineering at the Department of Informatics, Modeling, Electronics, and Systems Engineering of the University of Calabria, Italy. He received his master's degree in Computer Engineering and a PhD degree in Computer and Systems Engineering from the University of Calabria, respectively in 2007 and 2012. Between 2008 and 2010 he was employed as researcher at the Wireless Sensor Networks Lab in Berkeley, California with focus on the development of domain-specific frameworks for Wireless Body Sensor Networks (BSNs). From 2016 to 2022, he has been Assistant Professor of Computer Engineering at the University of Calabria. Since 2020, he has the Italian ASN (Scientific National Habilitation) for Full Professor. He is a young foreign visiting scientist at the Shenzhen Institutes of Advanced Technology, Chinese Academy of Sciences. He is the co-founder and CTO of the health-care sector at SenSysCal S.r.l., a spin-off of the University of Calabria, which develops innovative Internet-of-Things and sensor-based systems for mobile-health and home automation. His research activities include high-level programming methodologies and frameworks for Wearable Computing Systems (WCS), Collaborative WCS, pattern recognition and machine learning algorithms on physiological signals, human activity recognition, ECG analysis, emotion recognition, and Internet-of-Things modeling and simulation techniques. He has authored more than 100 indexed international publications (including over 30 papers in journals ranked Q1 by Scimago and one authored book), with 4600+ citations on Google Scholar and an H-index equal to 31. He is an associate member of the Technical Committee on Wearable Biomedical Sensors and Systems. He is a member of IEEE, IEEE Young Professionals Italy Section, and IEEE SMC Society. He has been involved in national and international research projects with various roles, including Principal Investigator.
报告摘要
To address the ever-increasing deluge of data collected and processed by computing systems, there is a trend toward processing data as close as possible to their source (edge computing). Both the EU and Gartner predict that by 2025 around 80% of enterprise data will be generated and processed outside the traditional cloud. In fact, edge computing is becoming even more attractive with the advent of energy-efficient micro-servers and powerful embedded devices with significant storage and processing capabilities. Another driver toward a cloud-edge computing continuum is 5G, both as a client of continuum resources and as a communication service enabler.
Moreover, the advent of the cloud-edge computing paradigm aggravates the challenging task of managing the loose federation of heterogeneous and distributed resources, this time at an extreme scale, making the traditional human-in-the-loop management unrealistic. To achieve dynamic and flexible system and application management with minimal user involvement, the concept of autonomic computing systems was proposed a long time ago as “computing systems that can manage themselves given high-level objectives from administrators”. However, the scale, heterogeneity, high dynamicity, and intrinsic variability of the continuum yield such rule-based approaches insufficient.
The talk will address a machine learning-based, software agent-enabled autonomic, efficient, and adaptive end-to-end system management on the heterogeneous and dynamic edge-cloud continuum by using AI models. We will introduce the objectives and results of MLSysOps, a European-funded project delivering an AI-driven control and management framework that interfaces with off-the-shelf management mechanisms, and employ a hierarchical, distributed, explainable, and evolving AI architecture for autonomic system operation. We employ a multi-level hierarchical ML software agent approach, in which agents at the lower levels will be responsible for the training and evolution of ML models that control the local compute and storage nodes, whereas agents at the higher levels will coordinate higher-level AI-driven control and ML training purposes within a layer or between layers.